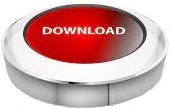
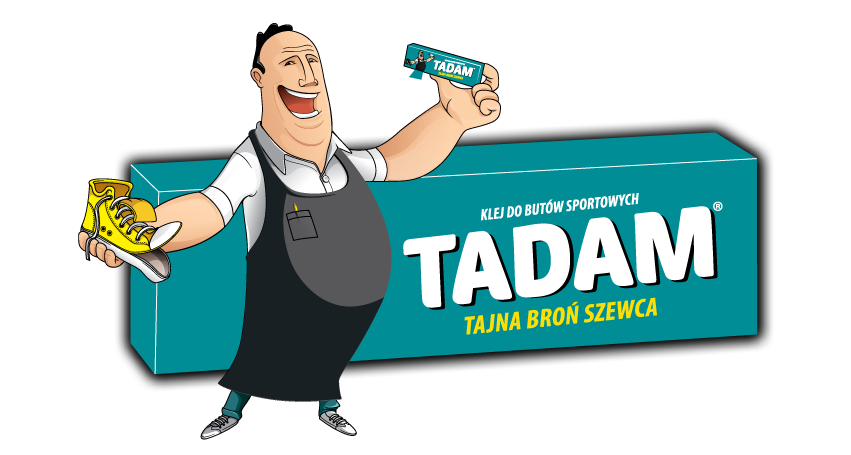
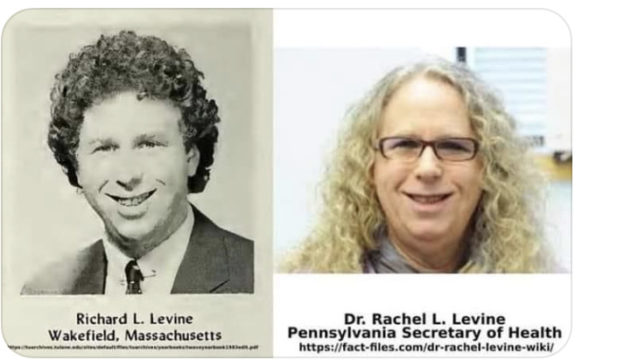
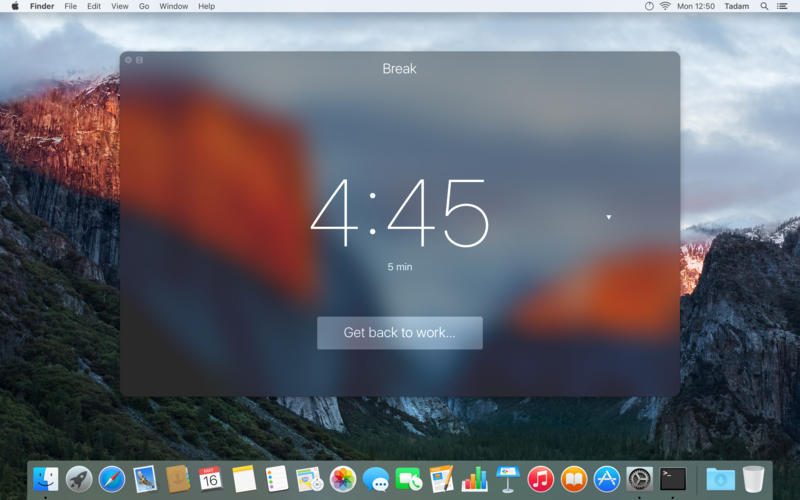
Chen W.-Y., Liu Y.-C., Kira Z., Wang Y.-C.F., Huang J.-B., A closer look at few-shot classification, in: ICLR, 2019.Szegedy C., Liu W., Jia Y., Sermanet P., Reed S., Anguelov D., Erhan D., Vanhoucke V., Rabinovich A., Going deeper with convolutions, in: CVPR, 2015, pp.Krizhevsky A., Sutskever I., Hinton G.E., Imagenet classification with deep convolutional neural networks, in: NeurlPS, 2012, pp.He K., Zhang X., Ren S., Sun J., Deep residual learning for image recognition, in: CVPR, 2016, pp.Krause J., Stark M., Deng J., Fei-Fei L., 3D object representations for fine-grained categorization, in: IEEE ICCV Workshops, 2013, pp.Van Horn G., Mac Aodha O., Song Y., Cui Y., Sun C., Shepard A., Adam H., Perona P., Belongie S., The inaturalist species classification and detection dataset, in: CVPR, 2018, pp.Li, Novel dataset for fine-grained image categorization: Stanford dogs, in: Proc. Belongie, The Caltech-UCSD Birds-200–2011 Dataset, Tech. Song K., Wei X., Shu X., Song R., Lu J., Bi-modal progressive mask attention for fine-grained recognition, IEEE Trans.Increasingly Specialized Generative Adversarial Network for fine-grained visual categorization, Knowl.-Based Syst.Cui Y., Song Y., Sun C., Howard A., Belongie S., Large scale fine-grained categorization and domain-specific transfer learning, in: CVPR, 2018, pp.The proposed approach is comparatively evaluated with state-of-the-art approaches in this field and demonstrates better performances consistently. The reconfigured model can train solely based on inter-class differences, which not only alleviates over-fitting, but also rediscovers more discriminating features. It is motivated by the fact that a large factor of the over-fitting under few-shot regime, comes from noises that appear in every layers of the activation. Secondly, a novel explainable-learning based procedure is integrated into the second phase to reconfigure the network for training on few samples, after which a deep adaptation procedure is conducted by simultaneously optimizing the feature extractor and the classification boundaries.
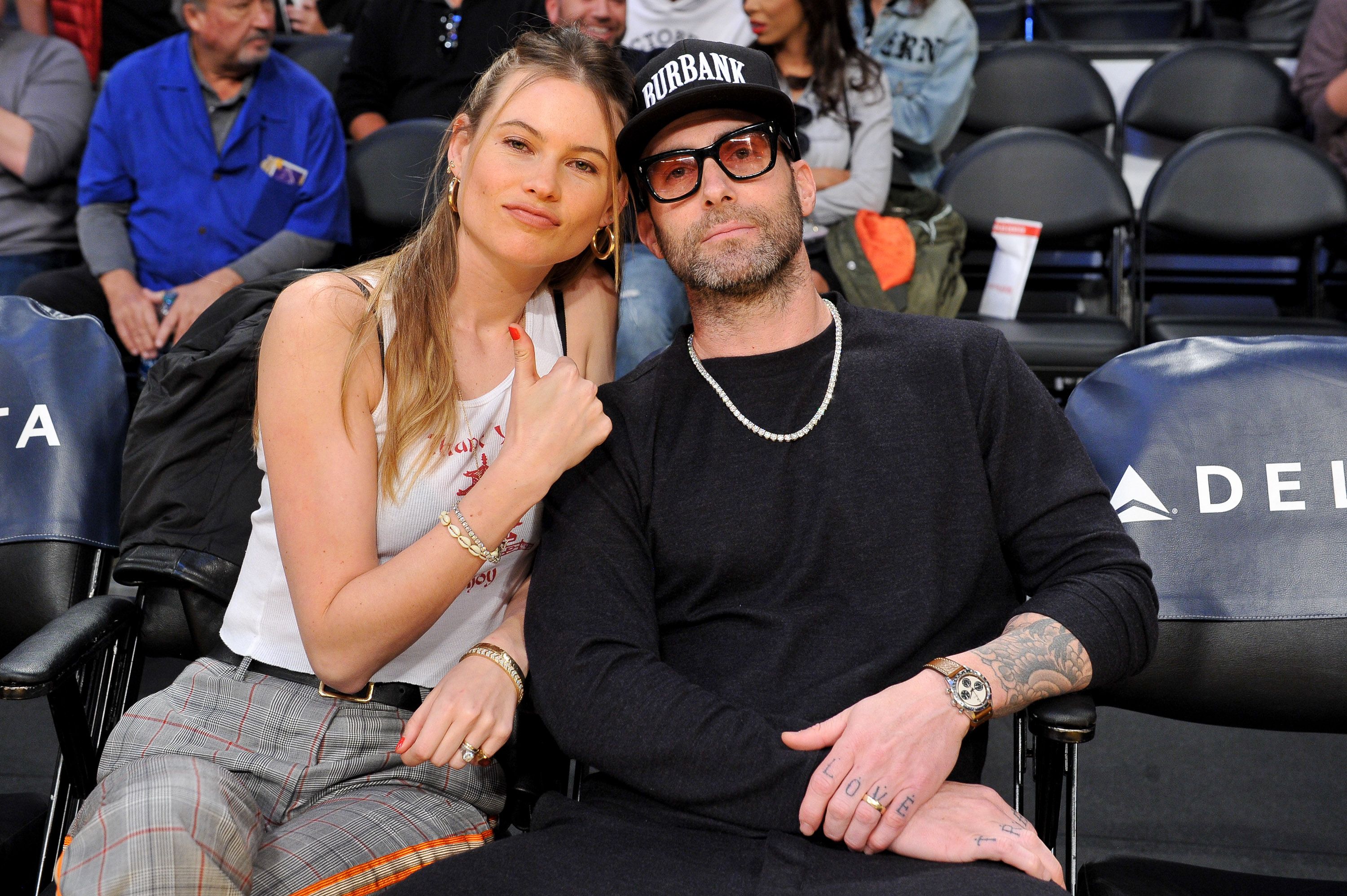
Firstly, a two-phase learning framework is proposed to facilitate task orientation and deep adaptation. As apposed to meta-learning which suffers limitations such as shallow adaptation, and traditional transfer learning which is not task-oriented, we propose a novel transfer learning approach which we refer to as Trans-transfer Learning (T2L). While transfer learning is extensively used in fine-grained visual categorization, these approaches generally does not work under few-shot regime. Fine-grained visual categorization requires the ability to distinguish categories with subtle differences, which is also a problem constantly burdened by collecting and labeling massive volume of samples.
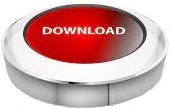